SEED Dataset
A dataset collection for various purposes using EEG signals
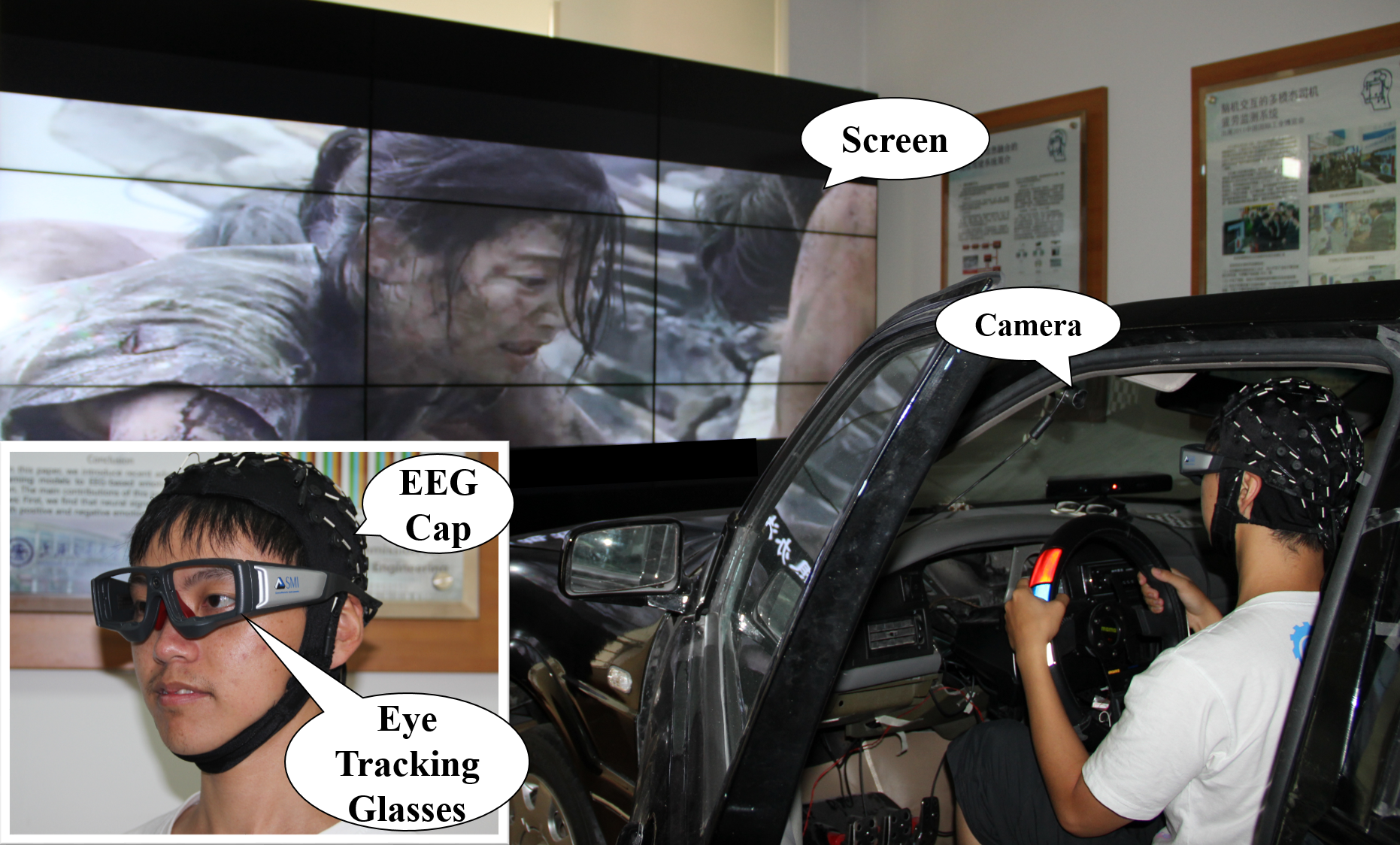
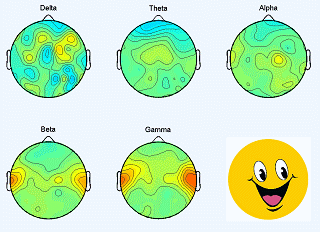
The SJTU Emotion EEG Dataset (SEED), is a collection of EEG datasets provided by the BCMI laboratory, which is led by Prof. Bao-Liang Lu and Prof. Wei-Long Zheng. The name is inherited from the first version of the dataset, but now we provide not only emotion but also datasets for other neuroscience research. As of September 2024, the cumulative number of applications and research institutions using SEED have reached more than 6340 and 2320, respectively. SEED series are open for the academic community and researchers from 93 countries are using our datasets. If you are interested in the datasets, take a look at the download page. NEWS: SEED-SD dataset has been released!
For a detailed description of the data files, please see the corresponding description page.
NEWS: SEED-DV dataset has been released!
For a detailed description of the data files, please see the corresponding description page.
The download access can be obtained with a request to the administrator.
NEWS: SEED-VII dataset has been released!
For a detailed description of the data files, please see the corresponding description page.
The download access can be obtained with a request to the administrator.
NEWS: SEED-VLA/VRW dataset has been released!
For a detailed description of the data files, please see the corresponding description page.
The download access can be obtained with a request to the administrator.
|
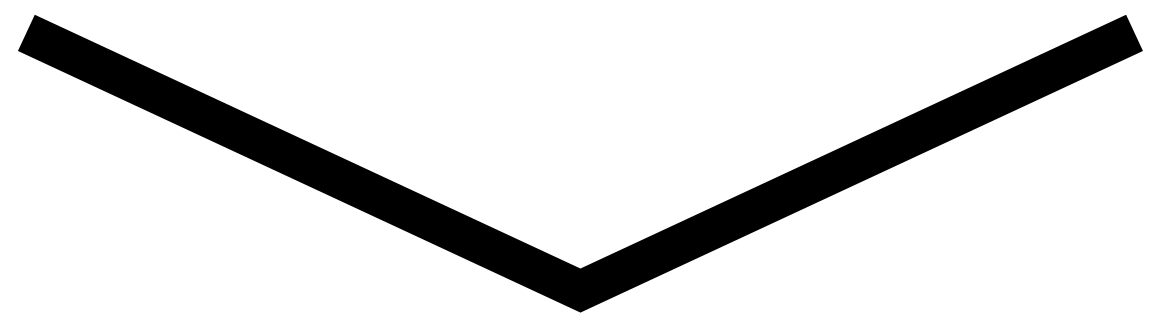
![]() |
![]() |
![]() |
The SEED dataset contains EEG and eye movement data of 12 subjects and EEG data of another 3 subjects. Data was collected when they were watching film clips. The film clips are carefully selected to induce different types of emotion, which are positive, negative, and neutral. Click here to know the details about the dataset.
![]() |
![]() |
![]() |
![]() |
The SEED-IV is an evolution of the original SEED dataset. The number of categories of emotions changes to four: happy, sad, fear, and neutral. In SEED-IV, we provide not only EEG signals but also eye movement features recorded by SMI eye-tracking glasses, which makes it a well-formed multimodal dataset for emotion recognition. Click here to know the details about the dataset.
![]() |
The SEED-VIG dataset is oriented at exploring the vigilance estimation problem. We built a virtual driving system, in which an enormous screen is placed in front of a real car. Subjects can play a driving game in the car, as if they are driving in the real-world environment. The SEED-VIG dataset is collected when the subjects drive in the system. The vigilance level is labeled with the PERCLOS indicator by the SMI eye-tracking glasses. Click here to know the details about the dataset. |
![]() |
![]() |
![]() |
![]() |
![]() |
The SEED-V is an evolution of the original SEED dataset. The number of categories of emotions changes to five: happy, sad, fear, disgust and neutral. In SEED-V, we provide not only EEG signals but also eye movement features recorded by SMI eye-tracking glasses, which makes it a well-formed multimodal dataset for emotion recognition. Click here to know the details about the dataset.
![]() |
![]() |
![]() |
![]() |
![]() |
![]() |
![]() |
The SEED-VII is a final version of the original SEED dataset. The number of categories of emotions changes to seven: happy, sad, fear, disgust, neutral, anger, and surprise. In SEED-VII, we provide not only EEG signals but also eye movement features recorded by Tobbi Pro Fusion eye-tracking devices, which makes it a well-formed multimodal dataset for emotion recognition. Click here to know the details about the dataset.
![]() |
![]() |
![]() |
The SEED-FRA dataset contains EEG and eye movement data of 8 French subjects with positive, negative and neutral emotional labels. Click here to know the details about the dataset.
![]() |
![]() |
![]() |
The SEED-GER dataset contains EEG and eye movement data of 8 German subjects with positive, negative and neutral emotional labels. Click here to know the details about the dataset.
![]() |
We developed SEED-VLA and SEED-VRW datasets for fatigue detection using EEG signals from lab and real-world driving. The lab setup included a simulated driving environment in a black car with a steering wheel and pedals, facing a large screen simulating various driving conditions. In real-world tests, participants in a Benben EV200 used a Logitech steering wheel and pedals, with vehicle speed limited for safety. Experiments aimed at inducing fatigue in controlled and naturalistic settings to assess vigilance through EEG. Click here to know the details about the dataset. |
![]() |
We developed the SEED-DV dataset for exploring decoding dynamic visual perception from EEG signals, recording 20 subjects EEG data when viewing 1400 video clips of 40 concepts. The dataset covers diverse natural videos of Land Animal, Water Animal, Plant, Exercise, Human, Nutural Scene, Food, Musical Instrument, and Transportation. Click here to know the details about the dataset. |
![]() |
We develop SEED-SD, a multimodal dataset comprising data from 40 participants. The dataset includes electroencephalography (EEG) and eye movement signals collected under three sleep conditions: sleep deprivation (SD), sleep recovery (SR), and normal sleep (NS). Each condition contains data corresponding to four basic emotions: happiness, sadness, fear, and neutral state. Click here to know the details about the dataset.
Acknowledgement
This work was supported in part by grants from the National Key Research and Development Program of China (Grant No. 2017YFB1002501), the National Natural Science Foundation of China (Grant No. 61272248 and No. 61673266), the National Basic Research Program of China (Grant No. 2013CB329401), the Science and Technology Commission of Shanghai Municipality (Grant No. 13511500200), the Open Funding Project of National Key Laboratory of Human Factors Engineering (Grant No. HF2012-K-01), the Fundamental Research Funds for the Central Universities, and the European Union Seventh Framework Program (Grant No. 247619).